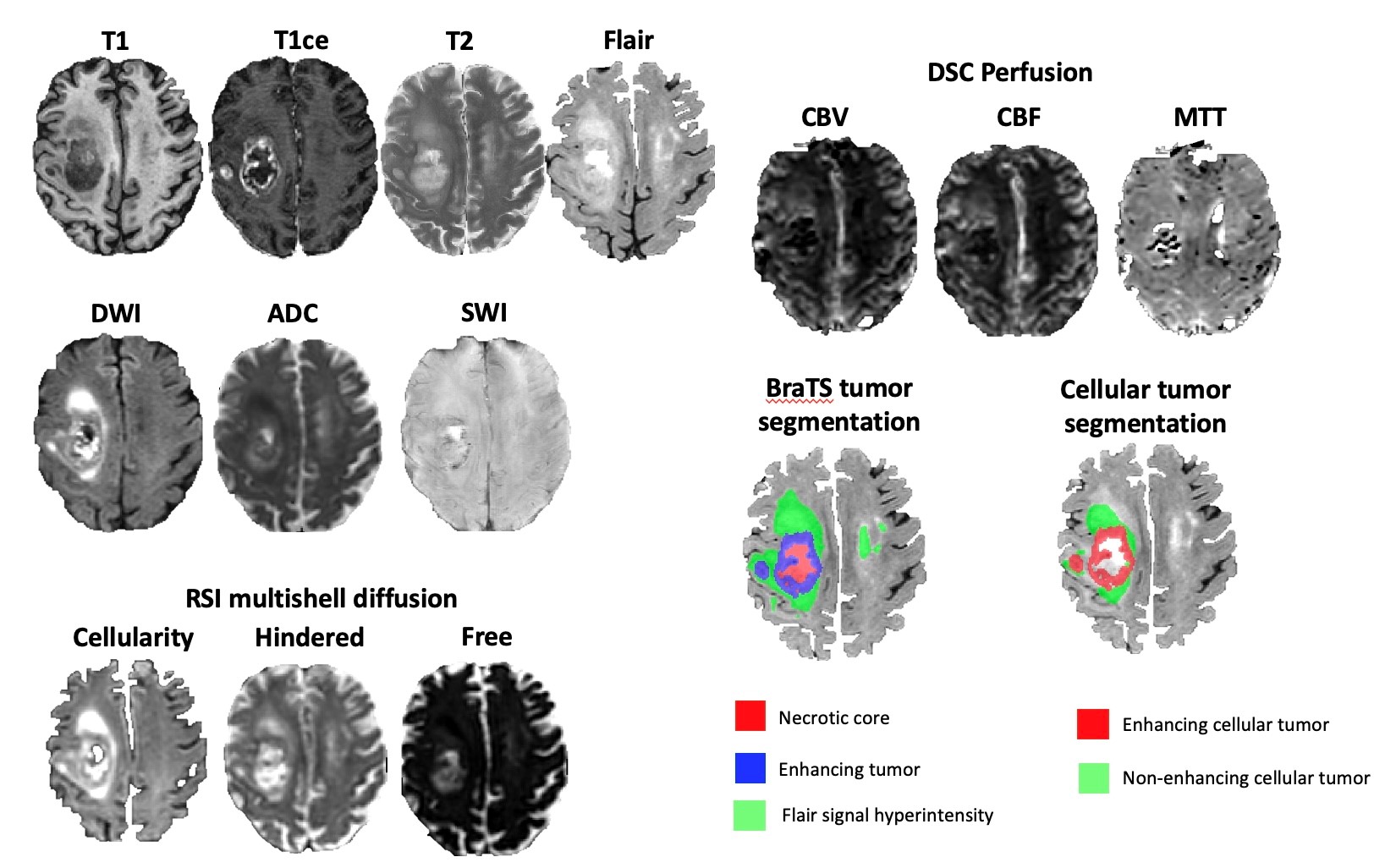
UCSD-PTGBM | The University of California San Diego annotated post-treatment high-grade glioma multimodal MRI dataset
DOI: 10.7937/fwv2-dt74 | Data Citation Required | 419 Views | Image Collection
Location | Species | Subjects | Data Types | Cancer Types | Size | Status | Updated | |
---|---|---|---|---|---|---|---|---|
Brain | Human | 178 | MR, Segmentation, Diagnosis, Demographic, Treatment, Follow-Up, Molecular Test | Glioblastoma | Clinical | Public, Complete | 2025/03/25 |
Summary
While there is a growing number of publicly available pre-operative MRI datasets for high-grade gliomas, very few post-operative datasets are available. Here we present the University of California San Diego post-operative high-grade glioma multimodal MRI (UCSD-PTGBM) dataset. The UCSD-PTGBM dataset includes 243 timepoints from 178 subjects (mean age 56 +/- 13 years [std], 116 men, 62 women) with histopathologically-proven high-grade gliomas who were imaged with an advanced brain tumor protocol on 3 Tesla MRI scanners. Sequences include standard 3D imaging, as well as multishell diffusion and perfusion imaging techniques (ASL and DSC), and neuroradiologist approved voxelwise tumor segmentations. The dataset also includes IDH mutation status and MGMT promoter methylation status, as well as overall survival and progression free survival information for several cases. We hope that researchers around the world will use these data to continue to improve analysis of post-operative MRI on high grade glioma patients. Patient population and demographic data: Patients diagnosed with high grade glioma from January 2010 to June 2022 from UCSD were identified from chart review of the institutional medical record. Inclusion criteria included post-treatment MRI with the multishell diffusion sequence Restricted Spectrum Imaging (RSI). The pathology database was searched to enrich the sample for cases with pathology proven progression and treatment-related changes. 244 unique patients (315 timepoints) were initially identified, but 54 time points were rejected due to missing MRI sequences and 18 time points were rejected due to inability to distinguish treatment-related changes from residual/recurrent tumor with confidence. The final dataset consisted of 178 patients (243 timepoints) with overall mean age 56 +/- 13 years [std], 116 men, 62 women. 192 timepoints had residual or recurrent tumor (45 pathology proven), while the remaining 51 timepoints had only post treatment changes (including 4 pathology-proven pseudoprogression (PsP), 9 clinically-confirmed PsP, 10 radiation necrosis, and 28 non-specific post-treatment changes). Overall survival (OS) and progression free survival (PFS) information is available for a subset of 94 patients (58 +/- 13 years, 67 men) with an MRI scan with RSI within 90 days following surgery. Image acquisition: Imaging protocol included pre- and postgadolinium 3D volumetric T1-weighted inversion recovery spoiled gradient-recalled sequences (TE/TR = 2.8/6.5 ms, TI = 450 ms, flip angle = 8 °, FOV = 24 cm, matrix = 0.93 x 0.93 x 1.2 mm) and a 3D T2- weighted FLAIR sequence (TE/TR = 126/6000 ms, TI = 1863 ms, FOV = 24 cm, matrix = 0.93 x 0.93 x 1.2 mm). For RSI, a single-shot pulsed-field gradient spin-echo echo-planar imaging sequence was used (TE/TR 1⁄4 96 ms/17 s; FOV 1⁄4 24 cm, matrix = 128×128×48; slice thickness = 2.5 mm) with 4 b-values (b = 0, 500, 1500, and 4000 s/mm2), and 6, 6, and 15 unique diffusion directions for each nonzero b-value, respectively (28 total volumes, ~8 min scan time). DSC perfusion MRI was performed using a gradient-echo echo-planar-imaging (EPI) sequence (TE/TR = 35/1600 ms; FA = 90°; slice thickness 5 mm with intersection gap of 1 mm; 22 axial slices; FOV = 25 cm; matrix = 96 × 96) 0.1 mmol/kg of gadobenate dimeglumine (Bracco Diagnostics) or gadobutrol (Bayer AG), was injected intravenously with an MR-compatible power injector at a rate of 2–3 mL/s through an antecubital angiocatheter, followed immediately by a 20-mL continuous saline flush. The multi-section image set was acquired every 1–2 s during the first pass of the contrast agent until 60 time points were obtained. Arterial Spin Labeling (ASL) was acquired using the standard GE protocol with in-line analysis. All patients were scanned on 3T clinical scanners (GE Signa Excite HDx and GE Discovery MR750 with 8-channel head coil) at 2 different institutions affiliated with UCSD. Image pre-processing: The RSI images were processed using a standard processing pipeline described in White et al [1] to derive cellularity maps. The diffusion signal in each voxel was fit using a linear mixture model of restricted and hindered water compartments with spherical and cylindrical geometries. The resulting spherically restricted water fraction formed the basis of our RSI cellularity maps. To maximize sensitivity and specificity to the spherically restricted water fraction, a beam-forming filter was applied to our cellularity maps to reduce residual signal contamination from cylindrically restricted and hindered water compartments. DSC images were processed using the Matlab DSC toolbox to compute Cerebral Blood Volume (CBV) using a leakage correction algorithm. Images from all modalities were registered and resampled to a 256x256x256 1 mm isotropic resolution MNI brain atlas. A brain mask was obtained from the FLAIR images using an inhouse custom nnUNet skull stripping network and was subsequently applied to the other modalities. Tumor segmentation: Benefit to Researchers: The UCSD-PTGBM will add on to a relatively small amount of publicly available post-operative high grade glioma datasets. The UCSD-PTGBM is the first dataset to include multishell diffusion MRI to separate tumor cellularity from free water (oedema) maps. It is also the first dataset to provide cellular tumor (both enhancing and non-enhancing) annotations by radiologists on top of standard (BraTS) tumor segmentation. The UCSD-PTGBM is one of very few datasets to include multiple perfusion techniques (DSC and ASL). It is also one of very few datasets to include progression free survival information on top of more conventional overall survival information. We believe that the UCSD-PTGBM dataset will extend radiology AI research to post-operative analysis and to the use of advanced imaging, as opposed to the more conventional preoperative standard multimodal MRI datasets used in the BraTS challenge. We look forward to the new discoveries that the UCSD-PTGBM will generate. References [1]. White NS, Leergaard TB, D’Arceuil H, Bjaalie JG, Dale AM. Probing tissue microstructure with restriction spectrum imaging: histological and theoretical validation. Hum Brain Mapp 2013;34(2):327–346. [2]. Baid U, Ghodasara S, Mohan S, et al. The RSNA-ASNR-MICCAI BraTS 2021 Benchmark on Brain Tumor Segmentation and Radiogenomic Classification. arXiv 2107.02314 [preprint] https://arxiv.org/abs/2107.02314. Posted July 5, 2021. Updated September 12, 2021. Accessed June 4, 2024.Abstract
Methods
Data Access
Version 1: Updated 2025/03/25
Title | Data Type | Format | Access Points | Subjects | License | Metadata | |||
---|---|---|---|---|---|---|---|---|---|
Images and Segmentations | MR, Segmentation | NIFTI | Download requires IBM-Aspera-Connect plugin |
136 | 184 | 4,047 | CC BY 4.0 | — | |
BraTS-GLI 2024 Test Data coming soon | MR, Segmentation | NIFTI | 42 | 59 | 1,322 | — | |||
Clinical Data | Diagnosis, Demographic, Treatment, Follow-Up, Molecular Test | XLSX | 136 | CC BY 4.0 | — |
Citations & Data Usage Policy
Data Citation Required: Users must abide by the TCIA Data Usage Policy and Restrictions. Attribution must include the following citation, including the Digital Object Identifier:
Data Citation |
|
Gagnon,L. Gupta,D., Mastorakos,G., White, N., V., Goodwill, McDonald, C., Beaumont, T., Conlin, C., Seibert, T., Nguyen, U., Hattangadi-Gluth, J., Kesari, S., Schulte,J., Piccioni, D., Schmainda,K., Farid, N., Dale, A., Rudie,J. (2025). The University of California San Diego annotated post-treatment high-grade glioma multimodal MRI dataset (UCSD-PTGBM) (version 1)[Dataset]. The Cancer Imaging Archive. https://doi.org/10.7937/fwv2-dt74 |
Acknowledgements
The SAIC Metadata Content Development Team identified existing or created new caDSR Common Data elements (CDEs) to describe the harmonized components of this dataset. The Metadata Content Development Team is supported by CBIIT under Task Order 140D0421F0008 from NCI.
Funding Sources
NIH SBIR grant 1R44NS120796-01A1
Related Publications
Publications by the Dataset Authors
The authors recommended the following as the best source of additional information about this dataset:
No other publications were recommended by dataset authors.
Research Community Publications
TCIA maintains a list of publications that leveraged this dataset. If you have a manuscript you’d like to add please contact TCIA’s Helpdesk.