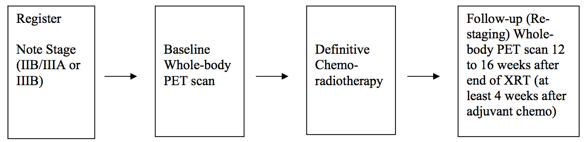
ACRIN-NSCLC-FDG-PET | ACRIN 6668
DOI: 10.7937/tcia.2019.30ilqfcl | Data Citation Required | 526 Views | 17 Citations | Image Collection
Location | Species | Subjects | Data Types | Cancer Types | Size | Status | Updated | |
---|---|---|---|---|---|---|---|---|
Lung | Human | 242 | Demographic, Diagnosis, Follow-Up, Measurement, Treatment, MR, NM, DX, SC, CR, CT, PT | Non-small Cell Lung Cancer | Clinical | Public, Complete | 2020/02/11 |
Summary
This was a multicenter clinical trial by the ACRIN Cooperative Group (now part of ECOG-ACRIN) and the RTOG Cooperative Group (now part of NRG) using FDG-PET imaging both pre- and post-chemoradiotherapy. The objective of the ACRIN 6668 multi-center clinical trial was to determine if the PET standardized uptake value (SUV) measurement from FDG-PET imaging shortly after treatment is a useful predictor of long-term clinical outcome (survival) after definitive chemoradiotherapy. Eligible patients were those older than 18 years with AJCC-criteria clinical stage IIB/III non-small cell lung carcinoma who were being planned for definitive concurrent chemoradiotherapy (inoperable disease). Additional information about the trial is available in the Study Protocol and Case Report Forms. Higher post-treatment tumor SUV (SUVpeak, SUVmax) is associated with worse survival in stage III NSCLC, although a clear SUV cutoff value for routine clinical use as a prognostic factor was uncertain. Later analyses found that larger pre-treatment metabolic tumor volumes (MTVs) were associated with significantly worse overall survival. Other secondary analyses found potentially predictive image texture biomarkers. Patients received conventional concurrent platinum-based chemoradiotherapy without surgery; post-radiotherapy consolidation chemotherapy was allowed. A baseline whole-body FDG-PET scan was performed prior to therapy. A second post-treatment whole-body FDG-PET scan occurred approximately 14 weeks after radiotherapy (at least 4 weeks after adjuvant chemotherapy). Pre-treatment FDG-PET scans were performed on ACRIN-qualified scanners. Post-treatment FDG-PET scans were required to be performed within 12–16 weeks after completion of therapy, using the same scanner as that used for the pre-treatment scans. Two sets of XLS spreadsheets (file set 1 and file set 2) are needed in order to obtain the entire clinical data set for this collection. The file sets are a random sample of ACRIN 6668 participants divided into 2 groups. Group 1/file set 1: a 75% random sample; Group 2: a 25% random sample initially held for testing/validating algorithms trained on the 75% sample. Positron Emission Tomography Pre- and Post-treatment Assessment for Locally Advanced Non-small Cell Lung Carcinoma
Primary Aim Findings:
Study Design Summary:
Data Access
Version 2: Updated 2020/02/11
Two sets of XLS spreadsheets (file set 1 and file set 2) are needed in order to obtain the entire clinical data set for this collection. The file sets are a random sample of ACRIN 6668 participants divided into 2 groups. Group 1/files set 1: a 75% random sample; Group 2: a 25% random sample initially held for testing/validating algorithms trained on the 75% sample.
Title | Data Type | Format | Access Points | Subjects | License | |||
---|---|---|---|---|---|---|---|---|
Images | MR, NM, DX, SC, CR, CT, PT | DICOM | Download requires NBIA Data Retriever |
242 | 950 | 3,377 | 497,367 | CC BY 3.0 |
Clinical, file set 1 | Demographic, Diagnosis, Follow-Up, Measurement, Treatment | XLS and ZIP | CC BY 3.0 | |||||
Clinical, file set 2 | Demographic, Diagnosis, Follow-Up, Measurement, Treatment | XLS and ZIP | CC BY 3.0 |
Additional Resources for this Dataset
The NCI Cancer Research Data Commons (CRDC) provides access to additional data and a cloud-based data science infrastructure that connects data sets with analytics tools to allow users to share, integrate, analyze, and visualize cancer research data.
- Imaging Data Commons (IDC) (Imaging Data)
- IDC Zenodo community dataset: Image segmentations produced by BAMF under the AIMI Annotations initiative
The following external resources are not hosted or supported by TCIA, but may be useful to researchers utilizing this collection.
- ClinicalTrials.gov entry about the Trial NCT00083083, “Positron Emission Tomography Pre- and Post-treatment Assessment For Locally Advanced Non-Small Cell Lung Carcinoma”
Citations & Data Usage Policy
Data Citation Required: Users must abide by the TCIA Data Usage Policy and Restrictions. Attribution must include the following citation, including the Digital Object Identifier:
Data Citation |
|
Kinahan, P., Muzi, M., Bialecki, B., Herman, B., & Coombs, L. (2019). Data from the ACRIN 6668 Trial NSCLC-FDG-PET (Version 2) [Data set]. The Cancer Imaging Archive. https://doi.org/10.7937/tcia.2019.30ilqfcl |
Detailed Description
Study Accrual:
Accrual began in June 2005 and ended in May 2009. Thirty-seven institutions accrued 250 patients to the study. Sixteen patients were ineligible, and eight patients did not have evaluable pretreatment PET, leaving 226 patients. Of these 226 patients, 173 had evaluable post- treatment PET, representing the analysis cohort for the primary end point. Data for 242 of the patients initially enrolled are available in TCIA.
Date Offsets:
All dates, like the visit date, are protected by presenting just the year; however, dates are also listed as offset days from the base date. The offset dates are used as a means of protecting patient information provided by the local sites in the original data, while allowing users to determine intervals between events. The standard DICOM date tags (i.e. birth dates, imaging study dates, etc.) have been de-identified so that all patients have a baseline study date of January 1, 1960. This falsified date represents the day patients were entered into trial database. The number of days between a subject’s longitudinal imaging studies are accurately preserved. A patient with a study performed on January 4, 1960 means the images were collected 3 days after the base date. For convenience, this calculation has been performed for all scans with the results inserted in DICOM tag (0012,0050) Clinical Trial Time Point ID . This means an imaging study that took place on January 4, 1960 would contain a value of “3” in tag (0012,0050).
Imaging Protocol:
Conventional modern equipment/techniques for FDG-PET (with or without PET/CT) were used in this study. Patients had to fast for 4 hours and have a blood glucose level less than 200 mg/dL before FDG injection. The FDG dose was not mandated; the recommended dose was 0.14 to 0.21 mCi/kg (approximately 10 to 20 mCi). Emission scanning began 50 to 70 minutes after FDG injection and included the body from upper/mid neck to proximal femurs. Acquisition times for emission and transmission scans were in accordance with the manufacturer’s recommendations.
Image Analysis:
PET scans were interpreted qualitatively and quantitatively by nuclear medicine physicians/radiologists at each institution, using standardized reporting forms to record the FDG uptake in the primary tumor, regional lymph nodes, and common sites of distant metastasis (ie, bones, adrenals, liver, contralateral lung). These local reviewers were provided with educational materials on image interpretation, specifically describing how to measure peak SUV (SUVpeak). However, formal demonstration of expertise was not mandated. SUVs for regions of interest (ROIs) were determined using two different metrics, maximum SUV (SUVmax) and SUVpeak. SUVmax represented the highest single-voxel SUV within the ROI. SUVpeak represented the mean SUV within a small circular ROI (0.75 to 1.5 cm in diameter) that encompasses the SUVmax voxel. (Thus, SUVpeak will always be lower than SUVmax.).
In addition to the institutional interpretations, pre- and post-treatment PET scans were centrally reviewed at ACRIN by an expert nuclear medicine physician with extensive experience in FDG-PET. A single dedicated workstation was used for this purpose, and SUVpeak was measured with an automated program in a circular ROI 1.5 cm in diameter. The central reader was blinded to clinical data and the institutional SUV measurements.
Outcomes:
Patients were observed for a minimum of 2 years (or until death) after completion of treatment in accordance with standard clinical practice. Non-protocol PET imaging was allowed but not mandated.
Overview of Clinical Data
The basic data flow for legacy ACRIN multi-center clinical trials was that all clinical information provided by the local imaging sites were contained in a series of forms. The form data submitted by local investigators to ACRIN during and after the trial, were manually encoded into the ACRIN CTMS (Clinical Trial Management System), and were cross-checked for accuracy by ECOG-ACRIN personnel. These ACRIN 6668 forms (see the ACRIN 6668 Data Forms page), filled out by the local sites, deliver information on imaging, clinical management of the patient and pathology/outcome variables, like dates of progression and survival, along with other critical information. The image data was initially anonymized while uploading from the local sites through TRIAD software and archived in a DICOM database at ACRIN.
After the trial accrual had ended, the clinical data was sent to the Brown statistical center, that is funded by NCI to provide support for ECOG-ACRIN clinical trials, specifically for analysis of the primary and sometimes secondary aims of the trial. The statisticians at Brown strip all the actual dates, names and other PHI from the CTMS data and create a .csv file for each form that has selected information useful for analysis of the trial data. A Form Description file detailing all the forms used in the study accompanies the .csv data files. Additionally, the accompanying Data Dictionary file lists each element for each form that has been selected for data retention along with a description of each form element.
Extracting clinical (non-imaging) data example:
Beginning with the Form Description.csv file, select the form with the desired information needed, such as form A0.csv the patient Eligibility/Registration form. Next, using the Data Dictionary.csv file, find the form elements listed for A0 (eg., A0exx, where xx is the form element number). The file lists the form number, variable name, its description or label, the type of data, and, when applicable, the option codes and corresponding text values (option code:description pairs like 1=’No’, 2=’Yes’; or 1=’Baseline’, 2=’Post treatment’) for each data element available from the form. In the example in Figure 2, the A0 form element 31d (A0e31d) reports the days between the base date and the day of surgery for the patient. In the corresponding A0.csv file column G lists the days between the base date and surgery for each patient.
In this example of extracting clinical data, the first step is to 1) find the form from the form list, 2) Find the desired element and description in the Data Dictionary and finally 3) extract the values from the .csv data file.
The procedure above is basically how the statisticians organized the selected data for export, but the structure of the data dictionaries and individual forms are different for each clinical trial.
Metadata
Much more information about this data set (ACRIN 6668 / RTOG 0235) can be found https://www.acrin.org/6668_protocol.aspx
Acknowledgements
This shared data set was provided in collaboration with the American College of Radiology Core Lab. Many thanks are due to the ACRIN 6668 trial team, and all the patients participating in the study. This study was supported by the American College of Radiology Imaging network (ACRIN), which received funding from the National Cancer Institute through UO1 CA080098, U01 CA21661, U01 CA32115, U01 CA190254, and R50 CA211270 (Muzi), under the American Recovery and Reinvestment ACT of 2009 (ARRA) and UO1 CA079778. Please see QIN ECOG-ACRIN Data Sharing page for an overview and list of other ECOG-ACRIN data collections available on TCIA.
Related Publications
Publications by the Dataset Authors
The authors recommended the following as the best source of additional information about this dataset:
Publication Citation |
|
Machtay, M., Duan, F., Siegel, B. A., Snyder, B. S., Gorelick, J. J., Reddin, J. S., Munden, R., Johnson, D. W., Wilf, L. H., DeNittis, A., Sherwin, N., Cho, K. H., Kim, S., Videtic, G., Neumann, D. R., Komaki, R., Macapinlac, H., Bradley, J. D., & Alavi, A. (2013). Prediction of Survival by [18F]Fluorodeoxyglucose Positron Emission Tomography in Patients With Locally Advanced Non–Small-Cell Lung Cancer Undergoing Definitive Chemoradiation Therapy: Results of the ACRIN 6668/RTOG 0235 Trial. In Journal of Clinical Oncology (Vol. 31, Issue 30, pp. 3823–3830). American Society of Clinical Oncology (ASCO). https://doi.org/10.1200/jco.2012.47.5947 |
No other publications were recommended by dataset authors.
Research Community Publications
TCIA maintains a list of publications that leveraged this dataset. If you have a manuscript you’d like to add please contact TCIA’s Helpdesk.