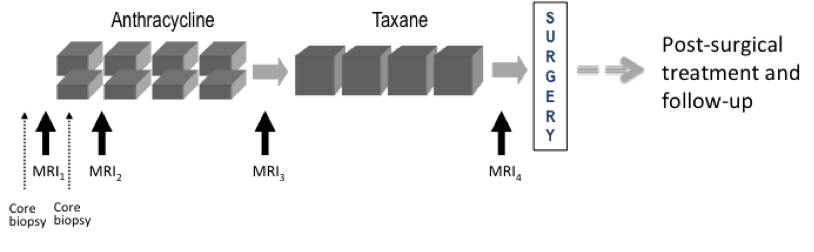
Breast-MRI-NACT-Pilot | Single site breast DCE-MRI data and segmentations from patients undergoing neoadjuvant chemotherapy
DOI: 10.7937/K9/TCIA.2016.QHsyhJKy | Data Citation Required | 1.1k Views | 18 Citations | Image Collection
Location | Species | Subjects | Data Types | Cancer Types | Size | Status | Updated | |
---|---|---|---|---|---|---|---|---|
Breast | Human | 64 | SEG, MR, Demographic, Diagnosis, Molecular Test, Follow-Up, Treatment, Measurement, Other | Breast Cancer | Clinical, Image Analyses | Public, Complete | 2023/08/07 |
Summary
This collection contains longitudinal DCE MRI studies of 64 patients undergoing neoadjuvant chemotherapy (NACT) for invasive breast cancer. This pilot study to investigate the use of serial DCE MRI examinations during neoadjuvant chemotherapy for invasive breast cancer recruited 68 patients with stage II or III locally advanced breast cancer enrolled between 1995 and 2002 in an institutional review board (IRB)-approved research protocol. All patients gave written informed consent to participate. All patients had confirmed breast cancer diagnoses based on histopathology of biopsy or surgical excision, and none had prior treatment with chemotherapy, surgery or radiation. All patients received pre-operative chemotherapy with four cycles of adriamycin-cytoxan administered every three weeks. A subset of 17 patients received additional weekly treatment with taxane after the Anthracycline regimen was completed. Three DCE-MRI scans were scheduled for all patients: MRI1 before treatment; MRI2 after one cycle of chemotherapy; MRI3 after completion of Anthracycline treatment and before surgery or further treatment. Subjects receiving Taxane were scheduled for an additional exam, MRI4, after completion of all chemotherapy treatment and before surgery. Figure 1. UCSF Pilot NACT study schema. Only an N=17 subset of patients received Taxane and MRI4 as a result of changes in standard of care during the course of the study. Note: Not all patients received core biopsies as indicated in this schema. Exclusions: Of the 68 patients, four have been excluded from this collection: one did not undergo surgery, one did not have a pre-surgery time-point MRI, one was rejected due to unanalyzable MR images, and one had metastatic disease prior to the completion of treatment. None of the excluded patients were from the 17 subject Taxane group. Three patients are included here but may be excluded at researchers' discretion due to treatment anomalies: a) Two subjects in the Taxane group (UCSF_BR_30 and UCSF_BR_53) did not complete the Taxane treatment, but were moved directly to surgery early and did not have the final MRI4 examination, and b) Patient UCSF_BR_27 declined standard-of-care post-surgery radiation and hormonal treatments. These cases are noted in the accompanying clinical information workbook. Endpoint data: Recurrence-free survival (RFS) was assessed for each patient at 6-month or 1-year intervals following surgery. For patients that recurred, length of RFS was defined as the time from initial surgery to either local or distant recurrence. Other clinical and endpoint data includes patient age, lesion characteristics including pretreatment tumor size, histologic type, pathologic size, tumor subtype, and lymph node involvement. These data are included as supplemental information for the collection in the accompanying clinical information workbook. Breast MRI was acquired on a 1.5-T scanner (Signa, GE Healthcare, Milwaukee, WI) using a bilateral phased array breast coil. The MR imaging protocol included a 3D localizer and a unilateral sagittal DCE acquisition. The DCE acquisition utilized a high spatial resolution, low temporal resolution, T1-weighted, fat-suppressed 3D fast gradient-recalled echo sequence developed for pre-surgical staging (TR/TE 8/4.2; flip angle 20 degrees; field of view 18-20 cm; acquisition matrix 256 x 192 x 60, section thickness 2 mm; spatial resolution 0.7 x 0.94 x 2.0 mm3). A minimum of three time points were acquired during each contrast-enhanced MRI protocol: a pre-contrast scan (t0), followed by 2 consecutive post-contrast time points: early (t1) and late (t2) phases. The gadopentetate dimeglumine contrast agent (Magnevist, Bayer HealthCare, Berlin, Germany), was injected at a dose of 0.1 mmol/kg of body weight (injection rate = 1.2 mL per second) followed by a 10 mL saline flush, with injection starting coincident with the start of the early t 1 phase acquisition. Imaging time was approximately 5 minutes per acquisition, resulting in effective early and late post-contrast time points of 2.5 minutes and 7.5 minutes from the start of the contrast injection, respectively, using standard k-space sampling. Fat suppression was performed using a frequency-selective inversion recovery preparatory pulse. This shared data set was provided by David Newitt, PhD and Nola Hylton, PhD from the Breast Imaging Research Program at UCSF. This work was supported by grants: Patient population
MRI acquisition
Data Access
Version 3: Updated 2023/08/07
Repaired a DICOM tag(0008,0005) to value “ISO_IR 100” in 79 series, affected ID: UCSF-BR-06, UCSF-BR-26, UCSF-BR-41, UCSF-BR-43, UCSF-BR-45, UCSF-BR-54, UCSF-BR-59, UCSF-BR-68.
Title | Data Type | Format | Access Points | Subjects | License | |||
---|---|---|---|---|---|---|---|---|
Images and Segmentations | SEG, MR | DICOM | Download requires NBIA Data Retriever |
64 | 189 | 2,224 | 98,936 | CC BY 3.0 |
Clinical and DFS Metadata | Demographic, Diagnosis, Molecular Test, Follow-Up, Treatment, Measurement | XLS | CC BY 3.0 | |||||
ISPY-1 DCE MRI Data Sharing Dictionary_v2 | Other | CC BY 3.0 |
Additional Resources for this Dataset
The NCI Cancer Research Data Commons (CRDC) provides access to additional data and a cloud-based data science infrastructure that connects data sets with analytics tools to allow users to share, integrate, analyze, and visualize cancer research data.
- Imaging Data Commons (IDC) (Imaging Data)
Citations & Data Usage Policy
Data Citation Required: Users must abide by the TCIA Data Usage Policy and Restrictions. Attribution must include the following citation, including the Digital Object Identifier:
Data Citation |
|
Newitt, D., & Hylton, N. (2016). Single site breast DCE-MRI data and segmentations from patients undergoing neoadjuvant chemotherapy (Version 3) [Data set]. The Cancer Imaging Archive. https://doi.org/10.7937/K9/TCIA.2016.QHsyhJKy |
Detailed Description
QIN BMMR Challenge
Subsets of this collection are being used as the training data set for the NCI Quantitative Imaging Network (QIN) Breast MRI Metrics of Response (BMMR) challenge. To download these subsets use the following links:
Download Link | Description |
---|---|
QIN_BMMR_Training DCE_Derived_HRknown | n=47 subject training set for bMMR Challenge, DCE series and derived maps and segmentations |
QIN_BMMR_Training DCEonly_HRknown | n=47 subject training set for BMMR Challenge, primary DCE series only |
QIN_BMMR_Training AllSeries_HRknown | n=47 subject training set for BMMR Challenge, all acquired and derived series |
QIN_BMMR_Training AllSeries_AllSubjects_n64 | n=64 training set for BMMR Challenge, all series all patients |
QIN_BMMR_Training DCEonly_AllSubjects_n64 | n=64 training set for BMMR Challenge, Original DCE series only, all patients |
QIN_BMMR_Training DCE_Derived_AllSubjects_n64 | n=64 training set for BMMR Challenge, DCE series and derived maps and segmentati, all patients |
BMMR_Training_Tissue_DSO_AllSubjects | Breast tissue segmentations for all studies in Breast-MRI-NACT-Pilot collection |
BMMR_Training_Tumor_DSO_AllSubjects | Breast enhancing tumor segmentations for all studies in Breast-MRI-NACT-Pilot collection |
Included image data
Original and time-of-scan derived images.
Original DCE images are contained in a single series for each study. For the majority of studies (174 of 198) this series contains only the 3 time-points described above. 12 studies have 1 additional post-contrast acquisition for a total of 4 phases, while 2 studies, UCSF_BR_36, visit 1, and UCSF_BR_42, visit 1, have 4 and 6 post-injection phases respectively.
NOTE: Study UCSF_BR_18, Visit 1, has two pre-contrast acquisitions and two post-contrast phases. Use of the 2nd pre-contrast phase for time-point t0 is recommended. All other studies have a single pre-contrast phase.
Other original image series present in the collection include 3-plane scout images for each study, and for some studies T2 weighted images and diffusion weighted images. In addition, some studies may contain derived images from the DCE series including subtraction images and projection images.
Tumor volumetric analysis images.
The primary predictor variable for the original study, functional tumor volume (FTV), was measured from contrast-enhanced images using the signal enhancement ratio (SER) method. Four derived series per study from this analysis are included in this collection:
Series ID
(x=original DCE series ID) |
Object Type | Series description |
---|---|---|
x1000 | MR | SER*1000 map1 |
x1001 | MR | PEearly map |
x2000 | Segmentation | PEearly segmentation for SER map calculation2 |
x2001 | Segmentation | Breast tissue segmentation for PEearly map calculation3 |
|
Further information on later NACT Breast Cancer MRI studies can be found at:
- ACRIN 6657 Protocol http://www.acrin.org/6657_protocol.aspx
- I-SPY TRIAL http://ncicb.nci.nih.gov/tools/translation_research/ispy (archive.org recovered deprecated page PDF per May 2023)
- CALGB 150007 http://www.cancer.gov/clinicaltrials/search/view?cdrid=69280&version=HealthProfessional (archive.org recovered deprecated page PDF per May 2023)
Metadata
Excel workbook with clinical data and disease free survival (DFS) data for the 64 patients in this study:
DICOM data dictionary defining fields included in the 0x0117 private group for PE/SER calculation parameters and results, and deduced DCE scan timing information. NOTE: Dictionary contains sections for attributes from the I-SPY 1 TRIAL which are not included in this pilot study.:
Acknowledgements
This shared data set was provided by David Newitt, PhD and Nola Hylton, PhD from the Breast Imaging Research Program at UCSF.
This work was supported by grants:
- National Institute of Health/National Cancer Institute: IUO1CA151235; RO1CA132870
- Susan G Komen: SAC110017
Related Publications
Publications by the Dataset Authors
The authors recommended the following as the best source of additional information about this dataset:
The Collection authors suggest the below will give context to this dataset:
- Partridge SC, Gibbs JE, Lu Y, Esserman LJ, Tripathy D, Wolverton DS, Rugo HS, Hwang ES, Ewing CA, Hylton NM. MRI measurements of breast tumor volume predict response to neoadjuvant chemotherapy and recurrence-free survival. AJR Am J Roentgenol. 2005 Jun;184(6):1774-81. PubMed PMID: 15908529. doi: 10.2214/ajr.184.6.01841774
- Li KL, Partridge SC, Joe BN, Gibbs JE, Lu Y, Esserman LJ, Hylton NM. Invasive breast cancer: predicting disease recurrence by using high-spatial-resolution signal enhancement ratio imaging. Radiology. 2008 Jul;248(1):79-87. PMID: 18566170. doi: 10.1148/radiol.2481070846
- Hattangadi J, Park C, Rembert J, Klifa C, Hwang J, Gibbs J, Hylton N. Breast stromal enhancement on MRI is associated with response to neoadjuvant chemotherapy. AJR Am J Roentgenol. 2008 Jun;190(6):1630-6. PubMed PMID: 18492917. doi: 10.2214/AJR.07.2533
- Li KL, Henry RG, Wilmes LJ, Gibbs J, Zhu X, Lu Y, Hylton NM. Kinetic assessment of breast tumors using high spatial resolution signal enhancement ratio (SER) imaging. Magn Reson Med. 2007 Sep;58(3):572-81. PubMed PMID: 17685424; PubMed Central PMCID: PMC4508009. doi: 10.1002/mrm.21361
- Jones EF, Sinha SP, Newitt DC, Klifa C, Kornak J, Park CC, Hylton NM. MRI enhancement in stromal tissue surrounding breast tumors: association with recurrence free survival following neoadjuvant chemotherapy. PLoS One. 2013 May 7;8(5):e61969. Print 2013. PMCID: PMC3646993. doi: 10.1371/journal.pone.0061969
Research Community Publications
TCIA maintains a list of publications that leveraged this dataset. If you have a manuscript you’d like to add please contact TCIA’s Helpdesk.
Previous Versions
Version 2: Updated 2016/04/16
deleted 4 duplicate DICOM series:
1.3.6.1.4.1.14519.5.2.1.7695.2311.274969196687715738621052447111
1.3.6.1.4.1.14519.5.2.1.7695.2311.790802609328661944330528970451
1.3.6.1.4.1.14519.5.2.1.7695.2311.869262000688001356814712906748
1.3.6.1.4.1.14519.5.2.1.7695.2311.914480028549654883806586724682
Title | Data Type | Format | Access Points | License | ||||
---|---|---|---|---|---|---|---|---|
Images | DICOM | Download requires NBIA Data Retriever |
||||||
Clinical and DFS Metadata | XLS | |||||||
ISPY-1 DCE MRI Data Sharing Dictionary_v2 |
Version 1: Updated 2016/01/30
Title | Data Type | Format | Access Points | License | ||||
---|---|---|---|---|---|---|---|---|
Images | DICOM | Download requires NBIA Data Retriever |
||||||
Clinical and DFS Metadata | XLS | |||||||
ISPY-1 DCE MRI Data Sharing Dictionary_v2 |