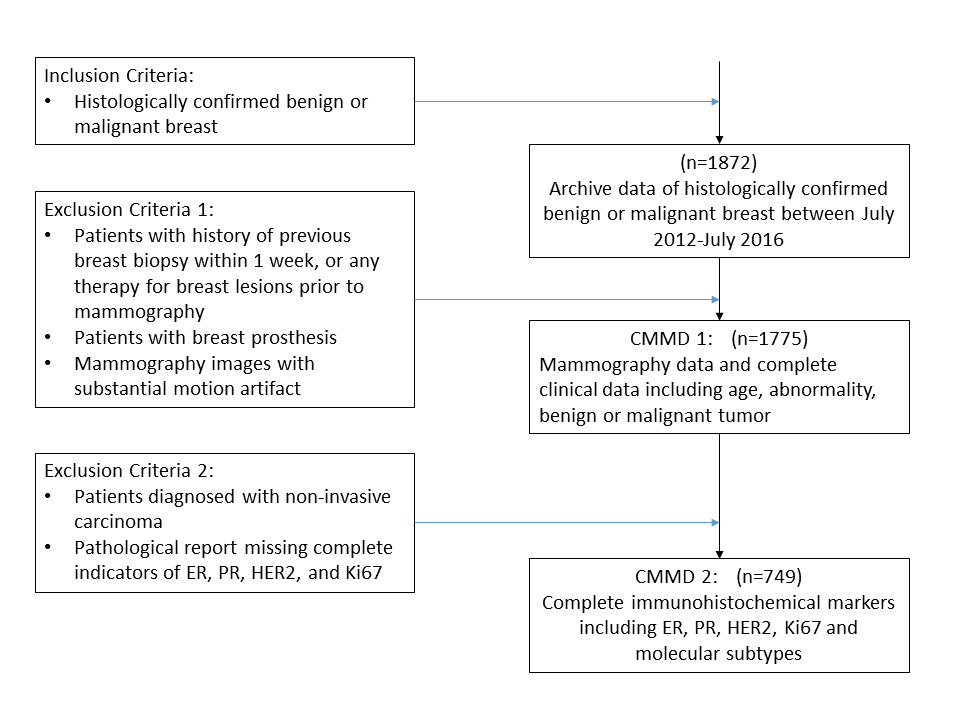
CMMD | The Chinese Mammography Database
DOI: 10.7937/tcia.eqde-4b16 | Data Citation Required | 2.2k Views | 16 Citations | Image Collection
Location | Species | Subjects | Data Types | Cancer Types | Size | Status | Updated | |
---|---|---|---|---|---|---|---|---|
Breast | Human | 1,775 | MG, Classification, Molecular Test, Demographic | Breast Cancer | Clinical | Public, Complete | 2021/04/06 |
Summary
Breast carcinoma is the second largest cancer in the world among women. Early detection of breast cancer has been shown to increase the survival rate, thereby significantly increasing patients' lifespans. Mammography, a noninvasive imaging tool with low cost, is widely used to diagnose breast disease at an early stage due to its high sensitivity. The recent popularization of artificial intelligence in computer-aided diagnosis creates opportunities for advances in areas such as (1) Computer-aided detection for locating suspect lesions such as mass and microcalcification, leaving the classification to the radiologist; and (2) Computer-aided diagnosis for characterizing the suspicious region of lesion and/or estimate its probability of onset; and (3) Findings of predictive image-based biomarkers by applying the computational methods to mine the potential relationships between image representation and molecular subtype, including luminal A, luminal B, HER2 positive, and Triple-negative. However, existing publicly available mammography databases are limited by small sample size, lack of diversity in patient populations, missing biopsy confirmations and unknown molecular sub-types. To help fill the gap, we built a database conducted on 1,775 patients from China with benign or malignant breast disease who underwent mammography examination between July 2012 and January 2016. The database consists of 3,728 mammographies from these 1,775 patients, with biopsy confirmed type of benign or malignant tumors. For 749 of these patients (1,498 mammographies) we also include patients' molecular subtypes. Image data were acquired on a GE Senographe DS mammography system.
Data Access
Version 1: Updated 2021/04/06
Title | Data Type | Format | Access Points | Subjects | License | |||
---|---|---|---|---|---|---|---|---|
Images | MG | DICOM | Download requires NBIA Data Retriever |
1,775 | 1,775 | 1,775 | 5,202 | CC BY 4.0 |
Clinical data | Classification, Molecular Test, Demographic | XLSX | 1,775 | CC BY 4.0 |
Additional Resources for this Dataset
The NCI Cancer Research Data Commons (CRDC) provides access to additional data and a cloud-based data science infrastructure that connects data sets with analytics tools to allow users to share, integrate, analyze, and visualize cancer research data.
- Imaging Data Commons (IDC) (Imaging Data)
Please note, it has been discovered that the hashes for the pixels of the following seem to be identical. TCIA does not know which is the “more correct” case for the files mentioned:
- D1-0202 (series UID ending with 31072, 1-1.dcm image) and D2-0284 (seriesUID ending with 98151, 1-1.dcm image)
- D1-0202 (series UID ending with 31072, 1-2.dcm image) and D2-0284 (seriesUID ending with 98151, 1-2.dcm image)
- D1-0202 (series UID ending with 31072, 1-3.dcm image) and D2-0284 (seriesUID ending with 98151, 1-3.dcm image)
- D1-0202 (series UID ending with 31072, 1-4.dcm image) and D2-0284 (seriesUID ending with 98151, 1-4.dcm image)
- D1-0808 (series UID ending with 62447, 1-1.dcm image) and D1-1292 (series UID ending with 65585, 1-1.dcm image)
Citations & Data Usage Policy
Data Citation Required: Users must abide by the TCIA Data Usage Policy and Restrictions. Attribution must include the following citation, including the Digital Object Identifier:
Data Citation |
|
Cui, Chunyan; Li Li; Cai, Hongmin; Fan, Zhihao; Zhang, Ling; Dan, Tingting; Li, Jiao; Wang, Jinghua. (2021) The Chinese Mammography Database (CMMD): An online mammography database with biopsy confirmed types for machine diagnosis of breast. The Cancer Imaging Archive. DOI: https://doi.org/10.7937/tcia.eqde-4b16 |
Detailed Description
- Mammography images were collected in .TIFF format and converted to DICOM.
- Clinical data are saved in .XLSX format. Note that for those rows where there exists BOTH a value for ID1 and ID2, TCIA image database stores ONLY the ID2 value as PatientID.
- For the D2-XXXX dataset, it is a dataset that only involves malignant tumors. Therefore, only one side of the clinical data is reasonable, such a situation shows that the other side is benign. We provided mammograms from both the left and right breast.
Acknowledgements
- The authors of this dataset thank the volunteers from the School of Computer Science and Engineering, South China University of Technology for assisting to tidy the clinical and imaging data. This work was supported by the grant from the National Natural Science Foundation of China (no.61771007).
- This work was partially supported by the Key-Area Research and Development of Guangdong Province under Grant (2020B010166002, 2020B1111190001), the National Natural Science Foundation of China (61472145, 61771007), Guangdong Natural Science Foundation (2017A030312008), and the Health & Medical Collaborative Innovation Project of Guangzhou City (201803010021, 202002020049).
- Harmonization of the components of this dataset, including into standard DICOM representation, was supported in part by the NCI Imaging Data Commons consortium. NCI Imaging Data Commons consortium is supported by the contract number 19X037Q from Leidos Biomedical Research under Task Order HHSN26100071 from NCI.
Related Publications
Publications by the Dataset Authors
The authors recommended the following as the best source of additional information about this dataset:
Publication Citation |
|
Cai, H., Huang, Q., Rong, W., Song, Y., Li, J., Wang, J., Chen, J., & Li, L. (2019). Breast Microcalcification Diagnosis Using Deep Convolutional Neural Network from Digital Mammograms. Computational and Mathematical Methods in Medicine, 2019, 1–10. https://doi.org/10.1155/2019/2717454 |
Publication Citation |
|
Wang, J., Yang, X., Cai, H., Tan, W., Jin, C., & Li, L. (2016). Discrimination of Breast Cancer with Microcalcifications on Mammography by Deep Learning. Scientific Reports, 6(1). https://doi.org/10.1038/srep27327 |
No other publications were recommended by dataset authors.
Research Community Publications
TCIA maintains a list of publications that leveraged this dataset. If you have a manuscript you’d like to add please contact TCIA’s Helpdesk.