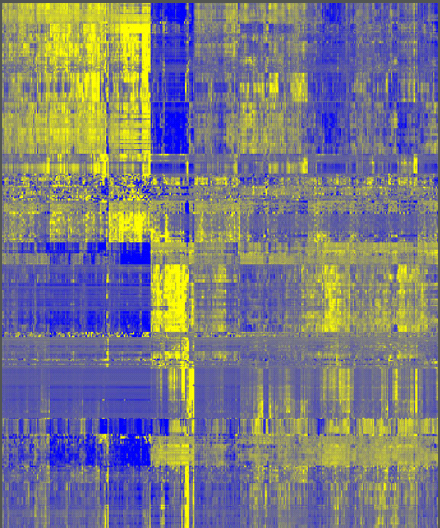
NSCLC-Radiomics-Genomics | NSCLC-Radiomics-Genomics
DOI: 10.7937/K9/TCIA.2015.L4FRET6Z | Data Citation Required | 2.1k Views | 28 Citations | Image Collection
Location | Species | Subjects | Data Types | Cancer Types | Size | Status | Updated | |
---|---|---|---|---|---|---|---|---|
Lung | Human | 89 | CT, Demographic, Measurement, Diagnosis, Molecular Test | Lung Cancer | Clinical, Genomics, Microarray | Public, Complete | 2014/07/02 |
Summary
This collection contains images from 89 non-small cell lung cancer (NSCLC) patients that were treated with surgery. For these patients pretreatment CT scans, gene expression, and clinical data are available. This dataset refers to the Lung3 dataset of the study published in Nature Communications.
In short, this publication applies a radiomic approach to computed tomography data of 1,019 patients with lung or head-and-neck cancer. Radiomics refers to the comprehensive quantification of tumour phenotypes by applying a large number of quantitative image features. In present analysis 440 features quantifying tumour image intensity, shape and texture, were extracted. We found that a large number of radiomic features have prognostic power in independent data sets, many of which were not identified as significant before. Radiogenomics analysis revealed that a prognostic radiomic signature, capturing intra-tumour heterogeneity, was associated with underlying gene-expression patterns. These data suggest that radiomics identifies a general prognostic phenotype existing in both lung and head-and-neck cancer. This may have a clinical impact as imaging is routinely used in clinical practice, providing an unprecedented opportunity to improve decision-support in cancer treatment at low cost.
The dataset described here (Lung3) was used to investigate the association of radiomic imaging features with gene-expression profiles. The Lung2 dataset used for training the radiomic biomarker and consisting of 422 NSCLC CT scans with outcome data can be found here: NSCLC-Radiomics. Other data sets in the Cancer Imaging Archive that were used in the same study published in Nature Communications: Head-Neck-Radiomics-HN1, NSCLC-Radiomics-Interobserver1, RIDER-LungCT-Seg.
Data Access
Version 1: Updated 2014/07/02
Title | Data Type | Format | Access Points | Subjects | License | Metadata | |||
---|---|---|---|---|---|---|---|---|---|
Images | CT | DICOM | Download requires NBIA Data Retriever |
89 | 89 | 89 | 13,482 | CC BY-NC 3.0 | View |
Lung3 clinical | Demographic, Measurement, Diagnosis, Molecular Test | XLS | CC BY-NC 3.0 | — |
Additional Resources for this Dataset
The following external resources have been made available by the data submitters. These are not hosted or supported by TCIA, but may be useful to researchers utilizing this collection.
The NCI Cancer Research Data Commons (CRDC) provides access to additional data and a cloud-based data science infrastructure that connects data sets with analytics tools to allow users to share, integrate, analyze, and visualize cancer research data.
- Imaging Data Commons (IDC) (Imaging Data)
Citations & Data Usage Policy
Data Citation Required: Users must abide by the TCIA Data Usage Policy and Restrictions. Attribution must include the following citation, including the Digital Object Identifier:
Data Citation |
|
Aerts HJWL, Rios Velazquez E, Leijenaar RTH, Parmar C, Grossmann P, Carvalho S, Bussink J, Monshouwer R, Haibe-Kains B, Rietveld D, Hoebers F, Rietbergen MM, Leemans CR, Dekker A, Quackenbush J, Gillies RJ, & Lambin P. (2015). Data From NSCLC-Radiomics-Genomics. The Cancer Imaging Archive. https://doi.org/10.7937/K9/TCIA.2015.L4FRET6Z |
Detailed Description
Corresponding microarray data acquired for the imaging samples are available at National Center for Biotechnology Information (NCBI) Gene Expression Omnibus (Link to GEO: http://www.ncbi.nlm.nih.gov/geo/query/acc.cgi?acc=GSE58661). The patient names used to identify the cases on GEO are identical to those used in the DICOM files on TCIA and in the clinical data spreadsheet.
Corresponding clinical data can be found here: Lung3.metadata.xls. DICOM patients names are identical in TCIA and clinical data file.
Related Publications
Publications by the Dataset Authors
The authors recommended the following as the best source of additional information about this dataset:
Publication Citation |
|
Aerts HJWL, Rios Velazquez E, Leijenaar RTH, Parmar C, Grossmann P, Carvalho S, Bussink J, Monshouwer R, Haibe-Kains B, Rietveld D, Hoebers F, Rietbergen MM, Leemans CR, Dekker A, Quackenbush J, Gillies RJ, & Lambin P. (2014) Decoding tumour phenotype by noninvasive imaging using a quantitative radiomics approach. Nature Communications 5, 4006 . https://doi.org/10.1038/ncomms5006 |
No other publications were recommended by dataset authors.
Research Community Publications
TCIA maintains a list of publications that leveraged this dataset. If you have a manuscript you’d like to add please contact TCIA’s Helpdesk.