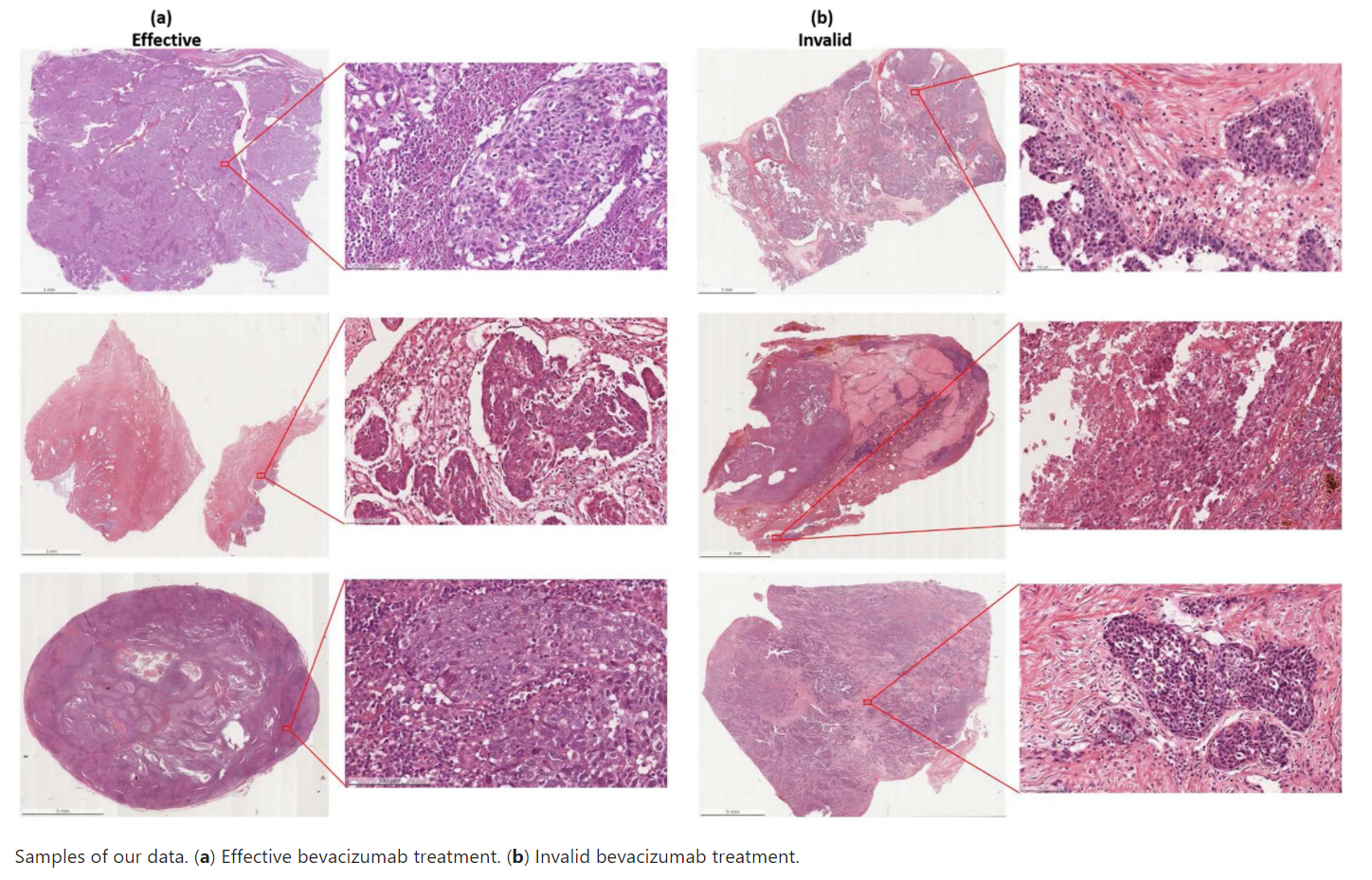
Ovarian Bevacizumab Response | A dataset of histopathological whole slide images for classification of Treatment effectiveness to ovarian cancer
DOI: 10.7937/TCIA.985G-EY35 | Data Citation Required | 1.1k Views | 8 Citations | Image Collection
Location | Species | Subjects | Data Types | Cancer Types | Size | Status | Updated | |
---|---|---|---|---|---|---|---|---|
Ovary | Human | 78 | Histopathology, Follow-Up, Measurement, Treatment, Exposure, Demographic, Diagnosis | Ovarian Cancer | Clinical | Public, Complete | 2023/04/26 |
Summary
Despite the progress made during the last two decades in the surgery and chemotherapy of ovarian cancer, more than 70% of advanced patients are with recurrent cancer and decease. Bevacizumab has been recently approved by FDA as a monotherapy for advanced ovarian cancer in combination with chemotherapy. Considering the cost, potential toxicity, and finding that only a portion of patients will benefit from these drugs, the identification of a new predictive method for the treatment of ovarian cancer remains an urgent unmet medical need. Prediction of therapeutic effects and individualization of therapeutic strategies are critical, but to the authors' best knowledge, there are no effective biomarkers that can be used to predict patient response to bevacizumab treatment for ovarian cancer. This dataset helps researchers to explore and develop methods to predict the therapeutic effect of patients with epithelial ovarian cancer to bevacizumab. The dataset consists of de-identified 288 hematoxylin and eosin (H&E) stained whole slides with clinical information from 78 patients. The slides were collected from the tissue bank of the Tri-Service General Hospital and the National Defense Medical Center, Taipei, Taiwan. Whole Slide Images (WSIs) were acquired with a digital slide scanner (Leica AT2) with a 20x objective lens. The dimension of the ovarian cancer slides is 54342x41048 in pixels and 27.34 x 20.66mm on average. The bevacizumab treatment is effective in 162 and invalid in 126 of the dataset. Ethical approvals have been obtained from the research ethics committee of the Tri-Service General Hospital (TSGHIRB No.1-107-05-171 and No.B202005070), and the data were de-identified and used for a retrospective study without impacting patient care. The clinicopathologic characteristics of patients were recorded by the data managers of the Gynecologic Oncology Center. Age, pre- and post-treatment serum CA-125 concentrations, histologic subtype, and recurrence, and survival status were recorded. A tumor, which is resistant to bevacizumab therapy, is defined as a measurable regrowth of the tumor or as a serum CA-125 concentration more than twice the value of the upper limit of normal during the treatment course for the bevacizumab therapy (i.e., the patient had the detectable disease or elevated CA-125 level following cytoreductive surgery combine with carboplatin/paclitaxel plus bevacizumab). A tumor, which is sensitive to bevacizumab therapy, is defined as no measurable regrowth of the tumor or as a serum CA-125 concentration under than twice the value of the upper limit of normal during the treatment course for the bevacizumab therapy. This dataset is further described in the following publications:
Data Access
Version 2: Updated 2023/04/26
Update: 2 files (414056O.svs and 414056P.svs) removed from folder e12.
1 file (220725D.svs) moved from folder in4 to e12.
Metadata spreadsheet “Final CA125 data.xlsx” updated with clinical information previously missing.
Title | Data Type | Format | Access Points | Subjects | License | |||
---|---|---|---|---|---|---|---|---|
Tissue Slide Images | Histopathology | SVS | Download requires IBM-Aspera-Connect plugin |
78 | 285 | CC BY 4.0 | ||
Clinical data: serum cancer antigen 125 data | Follow-Up, Measurement | XLSX | 79 | CC BY 4.0 | ||||
Clinical data: Final patient list | Follow-Up, Treatment, Exposure, Demographic, Diagnosis | XLSX | CC BY 4.0 |
Citations & Data Usage Policy
Data Citation Required: Users must abide by the TCIA Data Usage Policy and Restrictions. Attribution must include the following citation, including the Digital Object Identifier:
Data Citation |
|
Wang, C.-W., Chang, C.-C., Lo, S.-C., Lin, Y.-J., Liou, Y.-A., Hsu, P.-C., Lee, Y.-C., & Chao, T.-K. (2021). A dataset of histopathological whole slide images for classification of Treatment effectiveness to ovarian cancer (Ovarian Bevacizumab Response) (Version 2) [Data set]. The Cancer Imaging Archive. https://doi.org/10.7937/TCIA.985G-EY35 |
Detailed Description
Slides include 162 effective and 126 invalid images.
Acknowledgements
This research study is supported by the Ministry of Science and Technology of Taiwan, under a grant (MOST-108-2221-E-011-070, MOST109-2221-E-011-018-MY3, 110-2321-B-016 -002), Tri-Service General Hospital, Taipei, Taiwan (TSGH-C-108086, TSGH-D-109094, TSGH-D-110036, and TSGH-801GB111010), and Tri-Service General Hospital-National Taiwan University of Science and Technology (TSGH-NTUST-111-05).
Related Publications
Publications by the Dataset Authors
The authors recommended the following as the best source of additional information about this dataset:
Publication Citation |
|
Wang, C.-W., Chang, C.-C., Lee, Y.-C., Lin, Y.-J., Lo, S.-C., Hsu, P.-C., Liou, Y.-A., Wang, C.-H., & Chao, T.-K. (2022). Weakly Supervised Deep Learning for Prediction of Treatment Effectiveness on Ovarian Cancer from Histopathology Images. In Computerized Medical Imaging and Graphics (p. 102093). Elsevier BV. https://doi.org/10.1016/j.compmedimag.2022.102093 |
The Collection authors suggest the below will give context to this dataset:
- Wang, CW., Chang, CC., Khalil, M.A. et al. Histopathological whole slide image dataset for classification of treatment effectiveness to ovarian cancer. Sci Data 9, 25 (2022). https://doi.org/10.1038/s41597-022-01127-6
Research Community Publications
TCIA maintains a list of publications that leveraged this dataset. If you have a manuscript you’d like to add please contact TCIA’s Helpdesk.
Previous Versions
Version 1: Updated 2021/05/24
Title | Data Type | Format | Access Points | License | ||||
---|---|---|---|---|---|---|---|---|
Tissue Slide Images | SVS | Download requires IBM-Aspera-Connect plugin |
||||||
Clinical Data | XLS |