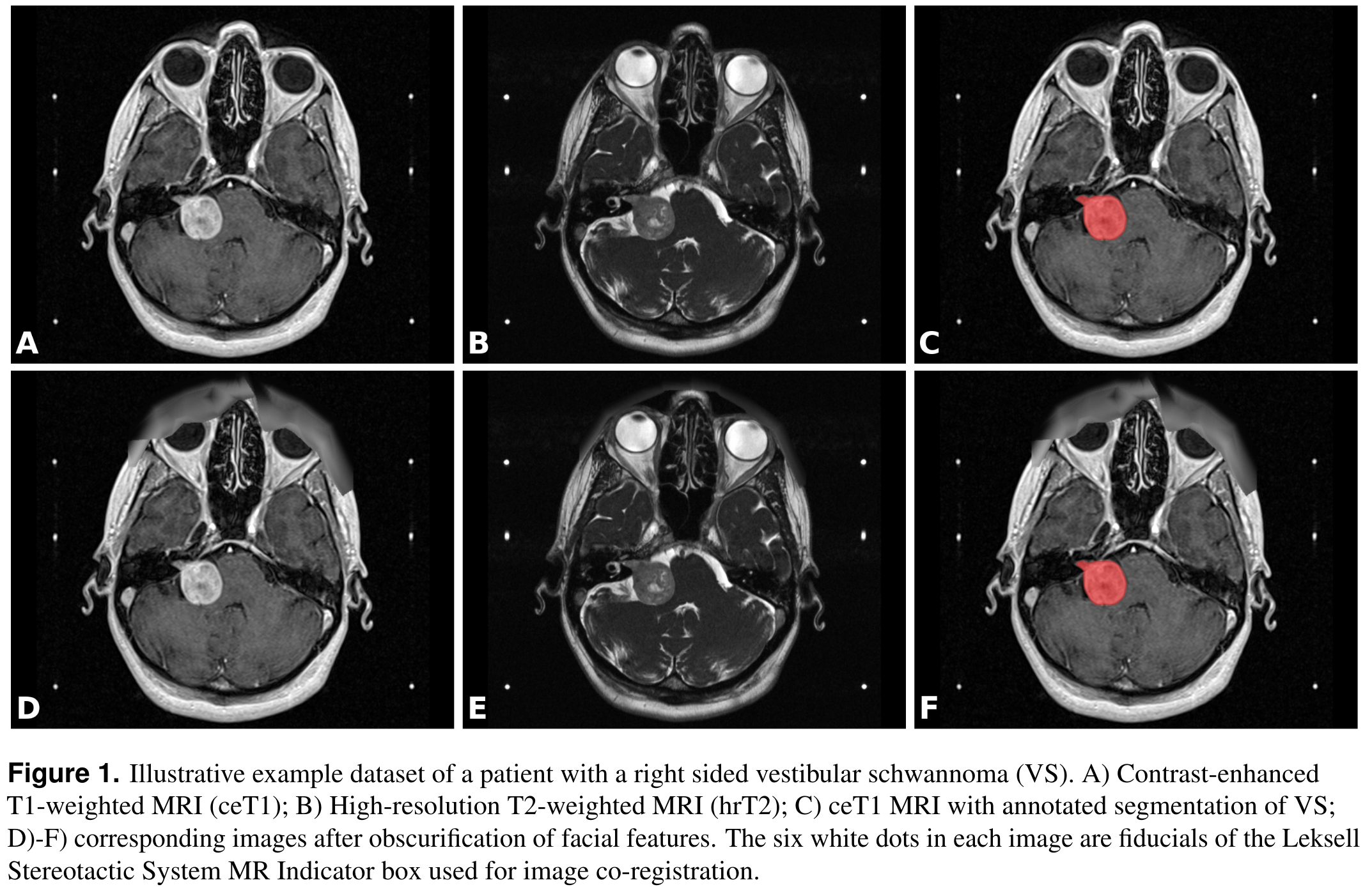
Vestibular-Schwannoma-SEG | Segmentation of Vestibular Schwannoma from Magnetic Resonance Imaging: An Open Annotated Dataset and Baseline Algorithm
DOI: 10.7937/TCIA.9YTJ-5Q73 | Data Citation Required | 1.2k Views | 9 Citations | Image Collection
Location | Species | Subjects | Data Types | Cancer Types | Size | Status | Updated | |
---|---|---|---|---|---|---|---|---|
Ear | Human | 242 | MR, RTDOSE, RTSTRUCT, RTPLAN, Other, Measurement, Radiomic Feature | Vestibular Schwannoma (non-cancer) | Image Analyses, Software/Source Code | Public, Complete | 2021/03/17 |
Summary
This collection contains a labeled dataset of MRI images collected on 242 consecutive patients with vestibular schwannoma (VS) undergoing Gamma Knife stereotactic radiosurgery (GK SRS). The structural images included contrast-enhanced T1-weighted (ceT1) images and high-resolution T2-weighted (hrT2) images. Each imaging dataset is accompanied by the patient’s radiation therapy (RT) dataset including the RTDose, RTStructures and RTPlan. Additionally, registration matrices (.tfm format) and segmentation contour lines (JSON format) are provided and described below. All structures were manually segmented in consensus by the treating neurosurgeon and physicist using both the ceT1 and hrT2 images. The value of this collection is to provide clinical image data including fully annotated tumour segmentations to facilitate the development and validation of automated segmentation frameworks. It may also be used for research relating to radiation treatment. Imaging data from consecutive patients with a single sporadic VS treated with GK SRS between the years of 2012 and 2018 were screened for the study. All adult patients older than 18 years with a single unilateral VS treated with GK SRS were eligible for inclusion in the study, including patients who had previously undergone operative surgical treatment. In total, 248 patients met these initial inclusion criteria including 51 patients who had previously undergone surgery. Patients were only included in the study if their pre-treatment image acquisition dataset was complete; 4 patients were thus excluded because of incomplete datasets and 2 further patients were excluded because the tumour was not completely contained in the image field of view. The images were obtained on a 32-channel Siemens Avanto 1.5T scanner using a Siemens single-channel head coil. All manual segmentations were performed using Gamma Knife planning software (Leksell GammaPlan, Elekta, Sweden) that employs an in-plane semiautomated segmentation method. Using this software, each axial slice was manually segmented in turn. Registration Matrices: For each subject and each modality there is a text file named inv_T1_LPS_to_T2_LPS.tfm or inv_T2_LPS_to_T1_LPS.tfm. The files specify affine transformation matrices that can be used to co-register the T1 image to the T2 image and vice versa. The file format is a standard format defined by the Insight Toolkit (ITK) library. The matrices are the result of the co-registration of fiducials of the Leksell Stereotactic System MR Indicator box into which the patient’s head is fixed during image acquisition. The localization of fiducials and co-registration was performed automatically by the LeksellGammaPlan software. Contours: For each subject and each modality there is a text file named contours.json. These contour files in the T1 and T2 folder contain the contour points of the segmented structures in JavaScript Object Notation (JSON) format, mapped in the coordinate frames of the T1 image and the T2 image, respectively. There can be small differences between the contour points of the RTSTRUCT and the contour points of the JSON files as explained in the following: Please see the github respository link which contains a script to organize the downloaded data into a more convenient folder structure and a script to perform co-registration based on the .tfm files and to convert the downloaded DICOM images and JSON contours into NIFTI format. Moreover, the repository contains an algorithm for automatic segmentation of VS with deep learning, adapted to this data set. The applied neural network is based on the 2.5D UNet described in "An artificial intelligence framework for automatic segmentation and volumetry of vestibular schwannomas from contrast-enhanced T1-weighted and high-resolution T2-weighted MRI" (Shapey, et al., 2021) and has been adapted to yield improved segmentation results. Our implementation uses MONAI, a freely available, PyTorch-based framework for deep learning in healthcare imaging (Project MONAI). This new implementation was devised to provide a starting point for researchers interested in automatic segmentation using state-of-the art deep learning frameworks for medical image processing. Registration matrices and JSON contours
In most cases, the tumour was segmented on the T1 image while the cochlea was typically segmented on the T2 image. This meant that some contour lines (typically for the tumour) were coplanar with the slices of the T1 image while others (typically for the cochlea) were coplanar with T2 slices. After co-registration, the (un-resampled) slices of the T1 and T2 image generally did not coincide; for example, due to different image position and, occasionally, slice thickness. Therefore, the combined co-registered contour lines were neither jointly coplanar with the T1 nor with the T2 image slices. Upon export of the segmentations in a given target space, the LeksellGammaPlan software interpolates between the original contour lines to create new slice-aligned contour lines in the target image space (T1 or T2). This results in the interpolated slice-aligned contour lines found in the RTSTRUCTs. In contrast, the contours in the JSON files were not interpolated after co-registration, and therefore describe the original (potentially off-target-space-slice) manual segmentation accurately.Related software
Data Access
Version 2: Updated 2021/03/17
Registration Matrices (.tfm) and Contours (JSON) were added to the dataset.
Title | Data Type | Format | Access Points | Subjects | License | Metadata | |||
---|---|---|---|---|---|---|---|---|---|
Images and Radiation Therapy Structures | MR, RTDOSE, RTSTRUCT, RTPLAN | DICOM | 242 | 242 | 1,936 | 48,582 | CC BY 4.0 | View | |
Directory names mapping to modality | Other | CSV | CC BY 4.0 | — | |||||
Registration Matrices | Other | TFM and ZIP | CC BY 4.0 | — | |||||
Contours | Measurement, Radiomic Feature | JSON and ZIP | CC BY 4.0 | — |
Additional Resources for this Dataset
The NCI Cancer Research Data Commons (CRDC) provides access to additional data and a cloud-based data science infrastructure that connects data sets with analytics tools to allow users to share, integrate, analyze, and visualize cancer research data.
- Imaging Data Commons (IDC) (Imaging Data)
Citations & Data Usage Policy
Data Citation Required: Users must abide by the TCIA Data Usage Policy and Restrictions. Attribution must include the following citation, including the Digital Object Identifier:
Data Citation |
|
Shapey, J., Kujawa, A., Dorent, R., Wang, G., Bisdas, S., Dimitriadis, A., Grishchuck, D., Paddick, I., Kitchen, N., Bradford, R., Saeed, S., Ourselin, S., & Vercauteren, T. (2021). Segmentation of Vestibular Schwannoma from Magnetic Resonance Imaging: An Open Annotated Dataset and Baseline Algorithm (version 2) [Data set]. The Cancer Imaging Archive. https://doi.org/10.7937/TCIA.9YTJ-5Q73 |
Acknowledgements
This work was supported by Wellcome Trust (203145Z/16/Z, 203148/Z/16/Z, WT106882), EPSRC (NS/A000050/1, NS/A000049/1) and MRC (MC_PC_180520) funding. Tom Vercauteren is also supported by a Medtronic/Royal Academy of Engineering Research Chair (RCSRF1819\7\34).
Related Publications
Publications by the Dataset Authors
The authors recommended the following as the best source of additional information about this dataset:
Publication Citation |
|
Shapey, J., Kujawa, A., Dorent, R., Wang, G., Dimitriadis, A., Grishchuk, D., Paddick, I., Kitchen, N., Bradford, R., Saeed, S. R., Bisdas, S., Ourselin, S., & Vercauteren, T. (2021). Segmentation of vestibular schwannoma from MRI, an open annotated dataset and baseline algorithm. In Scientific Data (Vol. 8, Issue 1). Springer Science and Business Media LLC. https://doi.org/10.1038/s41597-021-01064-w |
No other publications were recommended by dataset authors.
Research Community Publications
TCIA maintains a list of publications that leveraged this dataset. If you have a manuscript you’d like to add please contact TCIA’s Helpdesk.